by admin | Sep 17, 2021 | Software Testing, Blog |
For those looking to work in the field of QA and testing, it’s important to get the quality assurance skills and competencies required to become a first-class QA professional. QA engineers, in particular, require a combination of soft and hard skills that can help them work better alongside software developers. Here are some of those desirable qualities that every QA engineer should possess.
Broad Understanding of the Product
To test a product efficiently, a QA engineer needs to know it well enough to know how it should function normally. This skill may sound obvious, but a lot of QA engineers struggle with testing because they barely know the product and its capabilities. This skill is often taken for granted, especially if the QA engineer’s only goal is to just get it over with. This is the wrong mindset when testing products. You need to study the product thoroughly before you can perform accurate and impartial testing for it. The more you know the product, the better you’re able to test it and all its functions.
Proficiency in Bug Tracking, Ticketing, and Tests
A QA engineer needs to have a solid understanding of testing tools, opening and tracking tickets, and direct knowledge of a company’s QA process. The less time it takes for a company to train you, the more likely they are to hire you or promote you. This means you can already work independently and fulfill the functions of a QA engineer with little to no supervision. They also need to have a ‘customer-focused’ vision where they know exactly what the customer needs and how they think when using the product.
Avoiding QA Ping Pong
This is an industry term used by many QA professionals when the same ticket is being juggled between QA and developers several times. Such a situation can happen due to a number of reasons. Either the developer doesn’t want to admit their mistake and rejects the QA findings without verifying it, or the QA marks a ticket as failed as soon as the first bug occurs. Those two scenarios are very likely to happen, especially if both developers and QA engineers can’t get past their egos.
A good QA engineer will check whether any failed issues are affecting further use cases or not. This lessens the need for the unnecessary back and forth between developer and QA. If the fail issue doesn’t affect other use cases, then you can proceed so you can discover more bugs as early as possible.
Development Knowledge
Quality assurance requires knowledge about software development as well. While they don’t perform tasks as developers do, having knowledge of how the development process is done is a huge advantage, especially when testing software applications. Development capabilities also happen to be required to eventually code automated tests. If you want to make your job a little easier, you can create tools that could aid you in running an automated test. Also, knowing how to develop gives you an edge since you know what “dangerous” code is that requires focus when testing.
Conclusion
QA engineers basically understand what users need and help programmers build what is necessary for the product to become successful and easy to use. If you want to start a career in quality assurance, you better possess these skills in order for you to fulfill your role effectively.
Codoid is considered one of the top QA companies in the world today. With our team of QA experts passionate about software testing, we deliver a level of service that our competitors try to emulate but fail to do so. If you want to experience our QA services, partner with us today!
by admin | Aug 4, 2021 | Software Testing, Blog |
We’re truly living in a fast-paced digital world. Because of this, it’s much easier to miss out on golden opportunities if your products don’t reach the right consumers at an opportune time. Because of this, businesses need to act immediately when they receive feedback from their customers. By staying on top of your engagements with users, you can create changes in your campaigns and come closer to leading the pack in a saturated digital marketplace.
Automation testing is indeed an integral part of the software release cycle, especially since businesses can achieve more by integrating Continuous Integration and Continuous Deployment in the process. For this reason, working with a credible DevOps team and performance testing companies is a must if you want an agile-driven enterprise. With that being said, automation testing and DevOps work flawlessly to achieve this goal.
What is DevOps?
DevOps or Development and Operations cover a set of practices that limit software release cycles through continuous software delivery with excellent quality.
With DevOps, you get to create software enterprises that offer high-quality products and services that help accelerate your pace in the digital world and boost your ranking in the digital landscape.
How Can DevOps Speed Up Bug Fixes?
When you work with your consumers, you get to focus on integral parts of your product development that you won’t notice since you’re working on the backend. This is why consumer feedback is crucial since it plays a huge role in the betterment of your product.
But besides that, rolling out new product changes and updates frequently isn’t that easy. This is due to the complexities that may arise in updates that have been released on different devices, target geographies, and platforms. However, you can achieve a speedy rollout of updates by ensuring smooth product development, testing, and deployment strategy to eliminate bugs and glitches.
Seeing as manual testing isn’t enough to achieve these tasks, you need to cope with agile demands to help eliminate human errors and app bugs. With that being said, working with performance testing companies is crucial in developing a solid DevOps approach to fixing issues quickly.
Why Automation Testing and DevOps is a Winning Combination
There’s no doubt that integrating automation testing for web products can bring many benefits to your business. Especially now that you have a limited time to win the hearts of your consumers, you need to ensure that your product isn’t at their disposal.
Since you get to test your product at every stage, you get to boost your product’s performance and could help accelerate testing for the DevOps team, limiting the time it takes to revisit feedback and send it over to the development team.
With that being said, here’s why automation testing is crucial for DevOps:
- It provides consistent test results to help reap the benefits of an effective DevOps strategy;
- It improves your communications across different teams, eliminating reasons for delays;
- It helps produce faster test outcomes;
- Offers exceptional stability for product releases, which ultimately equates to great customer experience;
- Improves testing scalability and can focus on running different tests to help improve product quality.
The Bottom Line: Integrating Automation Testing and DevOps Strategies Can Help Improve Product Releases
Software enterprises are indeed walking on a thin rope, especially when you’re dealing with a fast-paced, ever-growing environment. With that, your software must be up to speed with these developments, and proper automation testing and DevOps strategies can help.
No matter the size of your company, it’s crucial that you consult with performance testing companies and experienced development teams to help provide you with test automation services that will be incorporated into your DevOps strategies.
Trust us — this will help boost your software capabilities and quality.
How Can Codoid Help You?
If you’re looking for performance testing companies, Codoid is here to help you.
We are an industry leader in QA, leading and guiding communities and clients with our passion for development and innovation. Our team of highly skilled engineers can help your team through software testing meetup groups, software quality assurance events, automation testing, and more.
Learn more about our services and how we can help you today!
by admin | Aug 16, 2021 | Software Testing, Blog, Latest Post |
In recent years organizations have invested significantly in structuring their testing process to ensure continuous releases of high-quality software. But all that streamlining doesn’t apply when artificial intelligence enters the equation. Since the testing process itself is more challenging, organizations are now in a dire need of a different approach to keep up with the rapidly increasing inclusion of AI in the systems that are being created. AI technologies are primarily used to enhance our experience with the systems by improving efficiency and providing solutions for problems that require human intelligence to solve. Despite the high complexity of the AI systems that increase the possibility of errors, we have been able to successfully implement our AI testing strategies to deliver the best software testing services to our clients. So in this AI Testing Tutorial, we’ll be exploring the various ways we can handle AI Testing effectively.
Understanding AI
Let’s start this AI Testing Tutorial with a few basics before heading over to the strategies. The fundamental thing to know about machine learning and AI is that you need data, a lot of data. Since data plays a major role in the testing strategy, you would have to divide it into three parts, namely test set, development set, and training set. The next step is to understand how the three data sets work together to train a neural network before testing your AI-based application.
Deep learning systems are developed by feeding several data into a neural network. The data is fed into the neural network in the form of a well-defined input and expected output. After feeding data into the neural network, you wait for the network to give you a set of mathematical formulae that can be used to calculate the expected output for most of the data points that you feed the neural network.
For example, if you were creating an AI-based application to detect deformed cells in the human body. The computer-readable images that are fed into the system make up the input data, while the defined output for each image forms the expected result. That makes up your training set.
Difference between Traditional systems and AI systems
It is always smart to understand any new technology by comparing it with the previous technology. So we can use our experience in testing the traditional systems to easily understand the AI systems. The key to that lies in understanding how AI systems differ from traditional systems. Once we have understood that, we can make small tweaks and adjustments to the already acquired knowledge and start testing AI systems optimally.
Traditional Software Systems
Features:
Traditional software is deterministic, i.e., it is pre-programmed to provide a specific output based on a given set of inputs.
Accuracy:
The accuracy of the software depends upon the developer’s skill and is deemed successful only if it produces an output in accordance with its design.
Programming:
All software functions are designed based on loops and if-then concepts to convert the input data to output data.
Errors:
When any software encounters an error, remediation depends on human intelligence or a coded exit function.
AI Systems:
Now, we will see the contrast of the AI systems over the traditional system clearly to structure the testing process with the knowledge gathered from this understanding.
Features:
Artificial Intelligence/machine learning is non – deterministic, i.e., the algorithm can behave differently for different runs since the algorithms are continuously learning.
Accuracy:
The accuracy of AI learning algorithms depends on the training set and data inputs.
Programming:
Different input and output combinations are fed to the machine based on which it learns and defines the function.
Errors:
AI systems have self-healing capabilities whereby they resume operations after handling exceptions/errors.
From the difference between each topic under the two systems we now have a certain understanding with which we can make modifications when it comes to testing an AI-based application. Now let’s focus on the various testing strategies in the next phase of this AI Testing Tutorial.
Testing Strategy for AI Systems
It is better not to use a generic approach for all use cases, and that is why we have decided to give specific test strategies for specific functionalities. So it doesn’t matter if you are testing standalone cognitive features, AI platforms, AI-powered solutions, or even testing machine learning-based analytical models. We’ve got it all covered for you in this AI Testing Tutorial.
Testing standalone cognitive features
Natural Language Processing:
1. Test for ‘precision’ – Return of the keyboard, i.e., a fraction of relevant instances among the total retrieved instances of NLP.
2. Test for ‘recall’ – A fraction of retrieved instances over the total number of retrieved instances available.
3. Test for true positives, True negatives, False positives, False negatives. Confirm that FPs and FNs are within the defined error/fallout range.
Speech recognition inputs:
1. Conduct basic testing of the speech recognition software to see whether the system recognizes speech inputs.
2. Test for pattern recognition to determine if the system can identify when a unique phrase is repeated several times in a known accent and whether it can identify the same phrase when repeated in a different accent.
3. Test how speech translates to the response. For example, a query of “Find me a place where I can drink coffee” should not generate a response with coffee shops and driving directions. Instead, it should point to a public place or park where one can enjoy coffee.
Optical character recognition:
1. Test the OCR and Optical word recognition basics by using character or word input for the system to recognize.
2. Test supervised learning to see if the system can recognize characters or words from printed, written or cursive scripts.
3. Test deep learning, i.e., check whether the system can recognize the characters or words from skewed, speckled, or binarized documents.
4. Test constrained outputs by introducing a new word in a document that already has a defined lexicon with permitted words.
Image recognition:
1. Test the image recognition algorithm through basic forms and features.
2. Test supervised learning by distorting or blurring the image to determine the extent of recognition by the algorithm.
3. Test pattern recognition by replacing cartoons with the real image like showing a real dog instead of a cartoon dog.
4. Test deep learning scenarios to see if the system can find a portion of an object in a large image canvas and complete a specific action.
Now we will be focusing on the various strategies for algorithm testing, API integration, and so on in this AI Testing Tutorial as they are very important when it comes to testing AI platforms.
Algorithm testing:
1. Check the cumulative accuracy of hits (True positives and True negatives) over misses (False positives and False negatives)
2. Split the input data for learning and algorithm.
3. If the algorithm uses ambiguous datasets in which the output for a single input is not known, then the software should be tested by feeding a set of inputs and checking if the output is related. Such relationships must be soundly established to ensure that the algorithm doesn’t have defects.
4. If you are working with an AI which involves neural networks, you have to check it to see how good it is with the mathematical formulae that you have trained it with and how much it has learned from the training. Your training algorithm will show how good the neural network algorithm is with its result on the training data that you fed it with.
The Development set
However, the training set alone is not enough to evaluate the algorithm. In most cases, the neural network will correctly determine deformed cells in images that it has seen several times. But it may perform differently when fed with fresh images. The algorithm for determining deformed cells will only get one chance to assess every image in real-life usage, and that will determine its level of accuracy and reliability. So the major challenge is knowing how well the algorithm will work when presented with a new set of data that it isn’t trained on.
This new set of data is called the development set. It is the data set that determines how you modify and adjust your neural network model. You adjust the neural network based on how well the network performs on both the training and development sets, this means that it is good enough for day-to-day usage.
But if the data set doesn’t do well with the development set, you need to tweak the neural network model and train it again using the training set. After that, you need to evaluate the new performance of the network using the development set. You could also have several neural networks and select one for your application based on its performance on your development set.
API integration:
1. Verify the input request and response from each application programming interface (API).
2. Conduct integration testing of API and algorithms to verify the reconciliation of the output.
3. Test the communication between components to verify the input, the response returned, and the response format & correctness as well.
4. Verify request-response pairs.
Data source and conditioning testing:
1. Verify the quality of data from the various systems by checking their data correctness, completeness & appropriateness along with format checks, data lineage checks, and pattern analysis.
2. Test for both positive and negative scenarios.
3. Verify the transformation rules and logic applied to the raw data to get the output in the desired format. The testing methodology/automation framework should function irrespective of the nature of the data, be it tables, flat files, or big data.
4. Verify if the output queries or programs provide the intended data output.
System regression testing:
1. Conduct user interface and regression testing of the systems.
2. Check for system security, i.e., static and dynamic security testing.
3. Conduct end-to-end implementation testing for specific use cases like providing an input, verifying data ingestion & quality, testing the algorithms, verifying communication through the API layer, and reconciling the final output on the data visualization platform with the expected output.
Testing of AI-powered solutions
In this part of the AI Testing Tutorial, we will be focusing on strategies to use when testing AI-powered solutions.
RPA testing framework:
1. Use open-source automation or functional testing tools such as Selenium, Sikuli, Robot Class, AutoIT, and so on for multiple purposes.
2. Use a combination of pattern, text, voice, image, and optical character recognition testing techniques with functional automation for true end-to-end testing of applications.
3. Use flexible test scripts with the ability to switch between machine language programming (which is required as an input to the robot) and high-level language for functional automation.
Chatbot testing framework:
1. Maintain the configurations of basic and advanced semantically equivalent sentences with formal & informal tones, and complex words.
2. Generate automated scripts in python for execution.
3. Test the chatbot framework using semantically equivalent sentences and create an automated library for this purpose.
4. Automate an end-to-end scenario that involves requesting for the chatbot, getting a response, and finally validating the response action with accepted output.
Testing ML-based analytical models
Analytical models are built by the organization for the following three main purposes.
Descriptive Analytics:
Historical data analysis and visualization.
Predictive Analytics:
Predicting the future based on past data.
Prescriptive Analytics:
Prescribing course of action from past data.
Three steps of validation strategies are used while testing the analytical model:
1. Split the historical data into test & train datasets.
2. Train and test the model based on generated datasets.
3. Report the accuracy of the model for the various generated scenarios as well.
All types of testing are similar:
It’s natural to feel overwhelmed after seeing such complexity. But as a tester, if one is able to see through the complexity, they will be able to that the foundation of testing is quite similar for both AI-based and traditional systems. So what we mean by this is that the specifics might be different, but the processes are almost identical.
First, you need to determine and set your requirements. Then you need to assess the risk of failure for each test case before running tests and determining if the weighted aggregated results are at a predefined level or above the predefined level. After that, you need to run some exploratory testing to find biased results or bugs as in regular apps. Like we said earlier, you can master AI testing by building on your existing knowledge.
With all that said, we know for a fact that an AI-based system provides a highly functional dynamic output with the same input when it is run again and again since the ML algorithm is a learning algorithm. Also, most of the applications today have some type of Machine Learning functionality to enhance the relationship of the applications with the users. AI inclusion on a much larger scale is inevitable as we humans will stop at nothing until the software we create has human-like functionalities. So it’s necessary for us to adapt to the progress of this AI revolution.
Conclusion:
We hope that this AI Testing Tutorial has helped you understand the AI algorithms and their nature that will enable you to tailor your own test strategies and test cases that cater to your needs. Applying out-of-the-box thinking is crucial for testing AI-based applications. As a leading QA company, we always implement the state of the art strategies and technologies to ensure quality irrespective of the software being AI-based or not.
by admin | Aug 6, 2021 | Software Testing, Blog, Latest Post |
Being good at any job requires continuous learning to become a habitual process of your professional life. Given the significance of DevOps in today’s day and age, it becomes mandatory for a software tester to have an understanding of it. So if you’re looking to find out what a software tester should know about DevOps, this blog is for you. Though there are several new terms revolving around DevOps like AIOps & TestOps, they are just the subsets of DevOps. Before jumping straight into the DevOps testing-related sections, you must first understand what DevOps is, the need for DevOps, and its principles. So let’s get started.
Definition of DevOps
“DevOps is about humans. DevOps is a set of practices and patterns that turn human capital into high-performance organizational capital” – John Willis. Another quote that clearly sums up everything about DevOps is from Gene Kim and it is as follows.
“DevOps is the emerging professional movement that advocates a collaborative working relationship between Development and IT Operations, resulting in the fast flow of planned work (i.e., high deploy rates), while simultaneously increasing the reliability, stability, resilience, and security of the production environment.” – Gene Kim.
The above statements strongly emphasize a collaborative working relationship between the Development and IT operations. The implication here is that Development and Operations shouldn’t be isolated at any cost.
Why do we need to merge Dev and Ops?
In the traditional software development approach, the development process would be commenced only if the requirements were captured fully. Post the completion of the development process, the software would be released to the QA team for quality check. One small mistake in the requirement phase will lead to massive reworks that could’ve been easily avoided.
Agile methodology advocates that one team should share the common goal instead of working on isolated goals. The reason is that it enables effective collaboration between businesses, developers, and testers to avoid miscommunication & misunderstanding. So the purpose here would be to keep everyone in the team on the same page so that they will be well aware of what needs to be delivered and how the delivery adds value to the customer.
But there is a catch when it comes to Agile as we are thinking only till the point where the code is deployed to production. Whereas, the remaining aspects like releasing the product in production machines, ensuring the product’s availability & stability are taken care of by the Operations team.
So let’s take a look at the kind of problems a team would face when the IT operations are isolated,
1. New Feature
Let’s say a new feature needs multiple configuration files for different environments. Then the dev team’s support is required until the feature is released to production without any errors. However, the dev team will say that their job is done as the code was staged and tested in pre-prod. It now becomes the Ops team’s responsibility to take care of the issue.
2. Patch Release
Another likely possibility is there might be a need for a patch release to fix a sudden or unexpected performance issue in the production environment. Since the Ops team is focused on the product’s stability, they will be keen to obtain proof that the patch will not impact the software’s stability. So they would raise a request to mimic the patch on lower environments. But in the meanwhile, end users will still be facing the performance issue until the proof is shown to the Ops team. It is a well-known fact that any performance issue that lasts for more than a day will most probably lead to financial losses for the business.
These are just 2 likely scenarios that could happen. There are many more issues that could arise when Dev and Ops are isolated. So we hope that you have understood the need to merge Dev and Ops together. In short, Agile teams develops and release the software frequently in lower environments. Since deploying in production is infrequent, their collaboration with Ops will not be effective to address key production issues.
When Dev + Ops = DevOps, new testing activities and tools will also be introduced.
DevOps Principles
We hope you’ve understood the need for DevOps by now. So let’s take a look at the principles based on which DevOps operate. After which we shall proceed to explore DevOps testing.
Eliminate Waste
Anything that increases the lead time without a reason is considered a waste. Waiting for additional information and developing features that are not required are perfect examples of this.
Build Quality In
Ensuring quality is not a job made only for the testers. Quality is everyone’s responsibility and should be built into the product and process from the very first step.
Create Knowledge
When software is released at frequent intervals, we will be able to get frequent feedback. So DevOps strongly encourages learning from feedback loops and improve the process.
Defer Commitment
If you have enough information about a task, proceed further without any delay. If not, postpone the decision until you get the vital information as revisiting any critical decision will lead to rework.
Deliver Fast
Continuous Integration allows you to push the local code changes into the master. It also lets us perform quality checks in testing environments. But when the development team pushes a bunch of new features and bug fixes into production on the day of release, it becomes very hard to manage the release. So the DevOps process encourages us to push smaller batches as we will be able to handle and rectify production issues quickly. As a result, your team will be able to deliver faster by pushing smaller batches at faster rates.
Respect People
A highly motivated team is essential for a product’s success. So when a process tries to blame the people for a failure, it is a clear sign that you are not in the right direction. DevOps lends itself to focus on the problem instead of the people during root cause analysis.
Optimise the whole
Let’s say you are writing automated tests. Your focus should be on the entire system and not just on the automated testing task. As a software testing company, our testers work by primarily focusing on the product and not on the testing tasks alone.
What is DevOps Testing?
As soon as Ops is brought into the picture, the team has to carry out additional testing activities & techniques. So in this section, you will learn the various testing techniques which are required in the DevOps process.
In DevOps, it is very common for you to see frequent delivery of any feature in small batches. The reason behind it is that if developers hand over a whole lot of changes for QA feedback, the testers will only be able to respond with their feedback in a day or two. Meanwhile, the developers would have to shift their focus towards developing other features.
So if any feedback is making a developer revisit the code that they had committed two or three days ago, then the developer has to pause the current work and recollect the committed code to make the changes as per the feedback. Since this process would significantly impact the productivity, the deployment is done frequently with small batches as it enables testers to provide quick feedback that makes it easy to revoke the release when it doesn’t go as expected.
A/B Testing
This type of testing involves presenting the same feature in two different ways to random end-users. Let’s say you are developing a signup form. You can submit two Signup forms with different field orders to different end-users. You can present the Signup Form A to one user group and the Signup Form B to another user group. Data-backed decisions are always good for your product. The reason why A/B testing is critical in DevOps is that it is instrumental in getting you quick feedback from end-users. It ultimately helps you to make better decisions.
Automated Acceptance Tests
In DevOps, every commit should trigger appropriate automated unit & acceptance tests. Automated regression testing frees people to perform exploratory testing. Though contractors are highly discouraged in DevOps, they are suitable to automate & manage acceptance tests. Codoid, as an automation testing company, has highly skilled automation testers, and our clients usually engage our test automation engineers to automate repetitive testing activities.
Canary Testing
Releasing a feature to a small group of users in production to get feedback before launching it to a large group is called Canary Testing. In the traditional development approach, the testing happens only in test environments. However, in DevOps, testing activities can happen before (Shift-Left) and after (Shift-Right) the release in production.
Exploratory Testing
Exploratory Testing is considered a problem-solving activity instead of a testing activity in DevOps. If automated regression tests are in place, testers can focus on Exploratory Testing to unearth new bugs, possible features and cover edge cases.
Chaos Engineering
Chaos Engineering is an experiment that can be used to check how your team is responding to a failure and verify if the system will be able to withstand the turbulent conditions in production. Chaos Engineering was introduced by Netflix in the year 2008.
Security Testing
Incorporate security tests early in the deployment pipeline to avoid late feedback.
CX Analytics
In classic performance testing, we would focus only on simulating traffic. However, we never try to concentrate on the client side’s performance and see how well the app is performing in low network bandwidth. As a software tester, you need to work closely with IT Ops teams to get various analytics reports such as Service Analytics, Log Analytics, Perf Analytics, and User Interaction Data. When you analyze the production monitoring data, you can understand how the new features are being used by the end-users and improve the continuous testing process.
Conclusion
So to sum things up, you have to be a continuous learner who focuses on methods to improve the product and deliver value. It is also crucial for everyone on the team to use the right tools and follow the DevOps culture. DevOps emphasizes automating the processes as much as possible. So to incorporate automated tests in the pipeline, you would need to know how to develop robust automated test suites to avoid false positives & negatives. If your scripts are useless, there is no way to achieve continuous testing as you would be continuously fixing the scripts instead. We hope that this has been an equally informative and enjoyable read. In the upcoming blog articles, we will be covering the various DevOps testing-related tools that one must know.
by admin | May 26, 2021 | Software Testing, Blog |
Open-source testing tools aid developers in determining functionality, regression, capacity, automation, and many other aspects of a mobile or desktop application. A software tool is open-source if its source code is available for free and if users can modify its original design.
Many open-source testing tools are available online—Robotil, Selenium, and JMeter are examples of open source testing tools, and they test different aspects of software use. QA and software testing services also use open-source tools; here are some reasons why open-source products could be a better option than a licensed tool.
Open-Source Tools Are Often More Stable
The most significant reason why you should choose open source tools is that they typically have large developer communities backing them, fueling innovation. With more developers or organizations using a tool, the more stable and mature it is. Selenium, a test automation tool for trying out actions in the browser, is one of the world’s most active open-source projects.
Selenium’s GitHub page shows hundreds of contributors and releases. In fact, Codoid has VisGrid, a GUI for Selenium Grid enabling a developer to create hubs and nodes for testing on a user interface instead of Command Prompt. Codoid released VisGrid in 2016, and it has since undergone 14 updates—the current version, VisGrid-1.14, integrates with Selenium 3.5.3.
Open-Source Tools Have No Vendor Lock-In
A common problem among companies that use proprietary databases, platforms, or software is vendor lock-in. When businesses choose a vendor with a lock-in period on their software, they cannot quickly move to an alternative without incurring much business disruption or expenses. Using open-source software is a workaround—since open source tools do not belong to only one vendor, companies do not have to deal with lock-in periods when using them.
Open-Source Tools Are Highly Customizable
If you want to use a single platform for many years, you need to ensure it will evolve with your needs. Companies would always need unique features that tools do not provide out of the box, so your QA tool should allow you to build a solution or adopt one from other developers.
It is why software testing companies use open-source tools. These software provide various extensions, plugins, custom commands, and other ways of modifying programs and applications to suit their clients’ needs. For example, Codoid has WelDree, a beginner-friendly, UI-based Cucumber feature executor. Some plugins or extensions are available on vendors’ websites or places like GitHub, while you can download others on forums and blogs.
Open-Source Tools Are Versatile
Software testing teams need to be fluent in various programming languages and know how to operate different platforms. The testing tools they use should be versatile and cover mobile apps, web apps, native apps, or hybrids. Open source tools are viable across platforms and languages, and they enable the versatility that software quality testers need. These tools have widespread adoption across organizations of all types and sizes.
Conclusion
Open source tools drive innovation and have plenty of support from developer communities. They are built on open standards, so you can customize open-source tools extensively. If you have needs unique to your company, using an open-source tool to build a custom solution is a great way of addressing these. Partnering with a performance testing company should ensure that your application works smoothly and efficiently.
Codoid is an industry-leading software testing company. We provide robust test automation solutions across cloud, mobile, and web environments, using Selenium, Appium, White Framework, Protractor, JMeter, and more. Contact us today for more information!
by admin | May 25, 2021 | Software Testing, Blog |
Test cases are documentations that consists of line-by-line instructions that define how testing has to be done. Test cases are very crucial when it comes to testing, as it acts as a well-defined guide for the testing team to validate if the application is free from bugs. Another important aspect is that test cases help to verify if the application meets the client’s requirements. So if a test case is written on the go without any planning or effort, it will result in huge losses, as a mistake here would act like a domino effect that impacts all the upcoming stages of testing. As one of the best software testing companies in the market, we have seen great results when effective test cases were implemented. So in this blog article, we will be focusing on how you can effectively write test cases.
What are the Headers that should be present in a Test Case?
Before we discuss on How to Write Effective Test Cases, let’s cover the basic aspects that every test case must have.
Name of the Project:
Whenever we write any test case, we should make sure to mention the name of the project to avoid any unnecessary confusion.
Module Name:
The software will usually be broken down into different modules so that they can be tested separately. So it is important to give each module a unique name so that different methods of testing can be assigned and implemented without any errors.
Reference Document:
A software requirements specification (SRS) or the Customer Requirement Specification (CRS) must be specified so that the testing team can test the application based on it.
Created By:
Every written piece of content would have an author and so make sure to mention the name of the person or the team that has created the test cases. Though it is not mandatory to include the designation of the person, it is recommended that you do so.
Test Case Created Date:
It goes without saying that when all the above information is vital, the date would be important as well. So make sure to specify the date that the test team should start testing, as it helps set a deadline.
Test Case ID:
Each and every Test Case should have a unique individual ID. It is like how each student has their own and unique identification number. It will help locate the test case if you want to reuse it or be helpful for any future references.
Test Scenario:
It acts as a descriptive guide that helps us understand each step. It also highlights the objective of the action that we are going to perform in the Test case.
Test Script (or) Steps:
Every step that should be carried out has to be mentioned under the Test Steps header. It is like explaining each step in an in-depth manner so that the testing test doesn’t miss out on any step resulting in deviations from the client requirement.
Test Data:
Mentioning what input should be given in each step is equally important while testing the application. It helps to keep the testing process on track without any missteps.
Expected Result:
The primary objective of any test is to verify if the expected or desired result is achieved at the end. The client requirement can be firmly established by properly defining the expected result to make the application error-free. Let’s take a look at an example to get a better understanding.
Example: If the user turns ON the fan switch, the Fan should rotate in an anticlockwise direction.
The expected result here is that the fan should rotate in the anticlockwise direction when it is switched on.
Actual Result:
But we cannot expect the result to be as expected in every scenario. That is why we have to make a note of the actual results we obtain while executing the test.
Example: In the same scenario as discussed above, it doesn’t mean that everything is working fine if the fan rotates. It must be verified according to the expectation and see if the fan rotates in the anticlockwise direction. If the fan rotates in the clockwise direction, we should make sure to make a record of it.
Status (or) Comment:
Once we have the expected result and the actual result, we will be able to come to a conclusion and decide if the application passed the test or not. Apart from updating the status, we can also add remarks about the result such as special scenarios and so on. We can also compare this to a student’s report sheet which denotes if the student has passed or failed and would have remarks based on the result as well.
What is the Difference Between Test Case and Test Scenario?
This could be a common question that arises in many minds when this topic is discussed. So we have created a tabular column to establish the key differences between a test case and a test scenario.
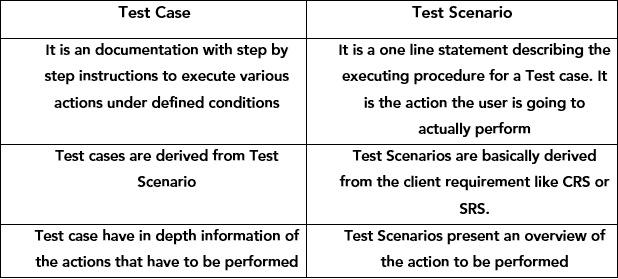
Sample Test Case:-
Now that we have gotten the basics out of the way, let’s dive into a full-fledged example and understand how to write effective test cases.
Test Scenario: A user has to send an email to someone else using their Gmail mobile application.
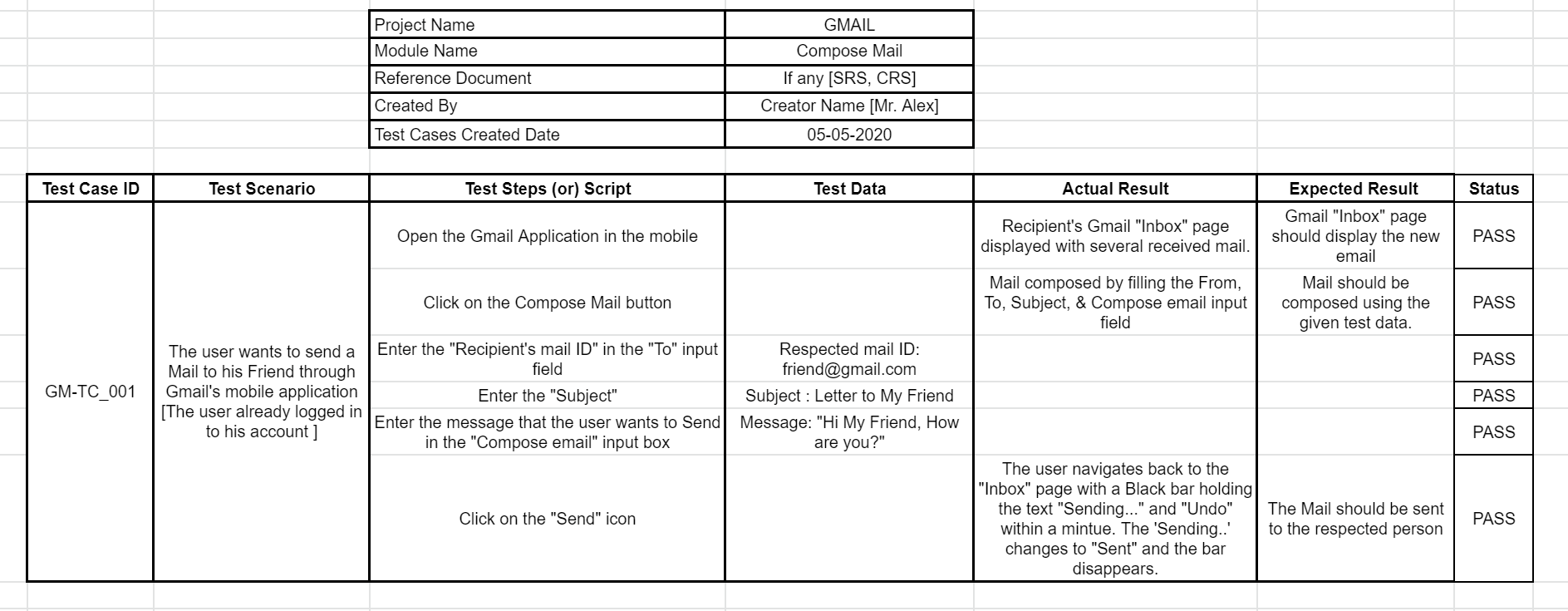
What are all the different types of Test Cases available?
Generally, test cases are classified into two main types,
i. Positive Test cases
ii. Negative Test cases
Positive Test cases:-
The Positive test cases do not differ much from the usual test cases, and so they are also known as formal test cases. A positive test case is used to very if the correct output is obtained when given the correct input.
Example:
Let’s say a user wants to transfer money through any UPI app, then he/she should find the correct recipient using their UPI ID or mobile number, enter the respected amount that they wish to transfer, and also enter the right UPI pin to complete the transaction. A positive test case would have the tester do the same actions by giving the correct input and seeing if everything works smoothly without any mishaps.
Negative Test cases:-
Negative Test cases are opposite to Positive test cases, as testers will provide an intentional negative or false input to make sure that the output is also negative. If we consider the same example we saw for positive test cases, the tester would try entering an incorrect UPI pin and see if the transaction fails. This is to make sure that only the authorized user will be able to operate the application, thereby increasing the security of the app. These tests are also called informal tests as they are not needed in every scenario, but they are pivotal in making sure that the application is secure.
Types of Positive Test Cases:-
Apart from the rare scenarios where negative test cases are used, positive test cases would be the more widely used preference. The Positive test cases can be further classified further based on the type of the project and the testing methods. But we will not be focusing on every type of test case as the other types are just subcategories of these 5 main types.
1. Functional Test cases
2. Integration Test cases
3. System Test Cases
4. Smoke Test cases
5. Regression Test Cases
Functional Test Cases:-
These test cases are written to check whether a particular functionality of the application is working properly or not.
Example:
If we take the same email sending example, then the test case that verifies if the send functionality is working correctly would be an apt example.
Integration Test Cases:-
These test cases are written to ensure that there is accurate data flow and database change across two combined modules or features.
Example:
The UPI money transaction example would fit well here too. During the transaction, the user enters the amount and enters the pin to complete the transaction. Once the transaction has been completed, the user will get a message about the deduction from their bank account as a result of the database change that has happened.
In this example, there is both data flow and database change between the two different modules.
System Test Cases:-
Earlier types saw us writing test cases only for a particular functionality or to see if the two modules work properly. But whenever the Testing environment is similar to the production environment, then the system test cases can be written to verify if the application is working correctly from its launch till the time it is closed. That is why this type of testing is also called end-to-end testing.
Example:
Here also we can take the same money transaction test case, but we have to test it starting right from the launch of the application to the successful transaction, and even until we receive the amount deduction message and have the transaction history updated in the user’s bank account.
Smoke Test Cases:-
Smoke tests that are also known as initial testing are very important in software testing as it acts like a preliminary test that every application should pass before extensive testing begins Smoke test cases should be written in a way that they check the critical functionalities of the software. We can then verify if the software is stable enough for further testing.
Example:
If we go back to the UPI payment app, then the testing team would have to test the application launch, account creation, and bank account addition before heading on to the transaction feature itself.
Regression Test Cases:-
Whenever the application gets any updates like UI updates, or new feature updates, the regression test cases come into play as they will be written based on the changes.
Example:
Let’s take an application most of us use on a daily basis, WhatsApp. Previously their testing team could have written test cases where the expected result could have been a list of emojis and the gif option being displayed when a user clicks on the Emoji option in the input text box.
But in one update, WhatsApp had introduced the stickers option. Since the new stickers would appear alongside the emojis and the gif option, the new test case would have been updated accordingly.
Guidelines on how to write effective test cases:
We have covered all the basic requisites that a test case must have and also the different types of test cases. This doesn’t necessarily mean that we can start writing perfect test cases right off the bat. So we will be covering a few important guidelines you should keep in your mind to write effective test cases.
1. CRS & SRS
The Testing team should be able to read and understand the Client requirements. But how is that made possible?
The client requirements for a project can be called a CRS or a Contract in simple terms. In this CRS document, the client will mention all their expectations about the software, such as how the software should perform and so on. This CRS document will be handled by the Business Analyst-(BA) of the company, and they will convert the CRS document into an SRS [System Requirement Specification] document.
The created SRS document has the functionality for each and every module and co-modules. Once the Business Analyst converts the CRS document into an SRS document they will provide the copy of the SRS document to the Testing and Development Teams. So the Testing team can read and understand the client requirement from the SRS.
2. Smoke Tests
Whenever we start writing the test cases, we should ensure to add smoke test cases to verify if the main functionality of the application is working.
3. Easy to Read
Writing easy-to-read test cases have advantages of their own. We can call it a success if a person who doesn’t have any technical knowledge can read the test cases and understand them clearly to work with the test cases.
4. Risk Evaluation
Writing extensive test cases that are not necessary should be avoided as it is a waste of resources. So proper risk evaluation has to be done prior to writing test cases so that the testing team can focus on the main requirement and core functionalities.
5. Format
The test cases always should start with either the application or the URL being launched, and it should end with the logging out option or closure of the application.
6. Avoid Assumptions
The Expected Result should be clearly captured for each and every test step, and all the features of the software (or) application should be tested without making any assumptions.
7. Dynamic values
Each and every step should not consist of multiple actions based on a single dynamic value. The dynamically changing values should not be hardcoded while writing the Test Cases. We have listed a few examples of such dynamic values.
Example:
Date, Time, Account Balance, Dynamically changing Game Slates, Reference Numbers, Points (or) Scores, etc.,
8. Dynamic Test Cases
But what should be done if we have to write a test case for a dynamically changing value? We can make use of common terms.
Example:
Let’s assume there is an online shopping site that is running a promotional campaign called ‘Happy New Year’ that provides its customers with a 20% off on their purchase. The dynamic value here is the campaign as it keeps changing in both name and value. So instead of writing a test case with the name of the one particular offer, it is better to use generic terms like ‘Promotional Campaign’
9. No Abbreviations
The Test cases should not be consist of any abbreviation (or) short forms. If in case the usage of an abbreviation is unavoidable, make sure to explain or define what it is in the test case. If not such open-ended information can lead to unnecessary mix-ups.
10. Optimal Distribution of Tests
A component level of verification should always happen only when you are writing functional test cases. You have to make sure that the same is not carried over when writing Integration and System Testing Test Cases. The Test Case document should cover all the types of the Test cases like Functional, Integration, System, and also Smoke Testing Test Cases.
How the test case helps the Testing team:
- Test case helps reduce the time and other resources required for testing.
- It helps to avoid test repetitions for the same feature of the application.
- It plays a major role in reducing the support maintenance cost of the software
- Even if we have to add a new member to the team during the course of the project, the new member will still be able to keep up with the team by reading the test cases.
- It helps to obtain maximum coverage of the application and fulfill the customer requirements
- The test cases help to ensure the quality of the software
- Test cases help us to think thoroughly and approach the process from as many angles as possible.
How to write effective test cases using the software?
The testing community has been writing test cases manually from the very beginning. But if you are looking for any software that reduces the time spent on writing test cases. You could take a look at the below test management systems,
Conclusion:
We hope you have enjoyed reading this blog. As a leading software testing service provider, we have always reaped the benefits of writing effective cases. But there is just no defined way to write test cases, as they are written in different formats depending on the project, the requirements, and the team involved. But we must always keep one point in our minds when we are thinking of how to write effective test cases, and that is to think from the point of view of the Client, Developer, End-User, and Tester. Then only we will be able to write quality test cases that will help us achieve 100% coverage.